

There are several reasons to look at modern data lakehouse architecture in order to drive sustainable data management practices. A data lakehouse architecture can also help companies ensure that data teams have the most accurate and updated data at their disposal for mission-critical machine learning, enterprise analytics initiatives, and reporting purposes. Data lakehouses are underpinned by a new open system architecture that allows data teams to implement data structures through smart data management features similar to data warehouses over a low-cost storage platform that is similar to the ones used in data lakes.Ī data lakehouse architecture allows data teams to glean insights faster as they have the opportunity to harness data without accessing multiple systems. It is an architectural approach for managing all data formats (structured, semi-structured, or unstructured) as well as supporting multiple data workloads (data warehouse, BI, AI/ML, and streaming).
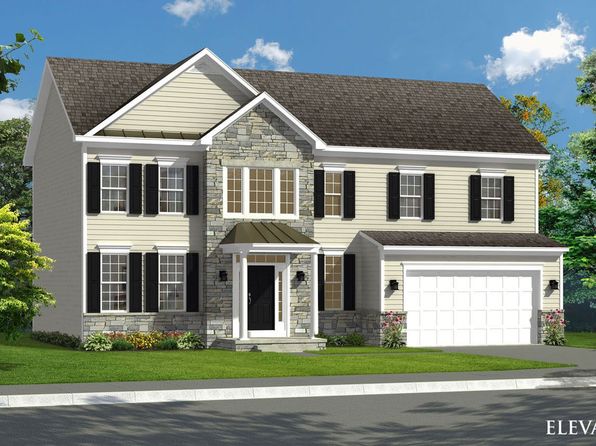
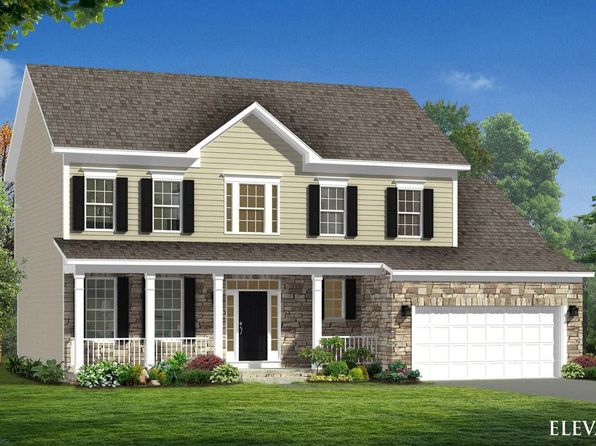
The data lakehouse: A brief overviewĪ data lakehouse is essentially the next breed of cloud data lake and warehousing architecture that combines the best of both worlds. Moreover, commercial warehouse data in proprietary formats increases the cost of migrating data.Ī data lakehouse addresses these typical limitations of a data lake and data warehouse architecture by combining the best elements of both data warehouses and data lakes to deliver significant value for organizations.

Advanced analytics limitations: Advanced machine learning applications such as PyTorch and TensorFlow aren’t fully compatible with data warehouses.Data governance: While the data in the data lake tend to be mostly in different file-based formats, a data warehouse is mostly in database format, and it adds to the complexity in terms of data governance and lineage.Poor maintainability: With data lakes and data warehouses, companies need to maintain multiple systems and facilitate synchronization which makes the system complex and difficult to maintain in the long run.Additionally, data stored in the warehouses is also harder to share with all data end-users within an organization. Vendor lock-in: Shifting large volumes of data into a centralized EDW becomes quite challenging for companies not only because of the time and resource required to execute such a task but also because this architecture creates a closed-loop causing vendor lock-in.Constantly changing datasets: The data stored in a data warehouse may not be as current as the data in a data lake which depends upon the data pipeline schedule and frequency.Each step can introduce failures and unwanted bugs affecting the overall data quality. It is not just a costly affair, but teams also need to employ continuous data engineering tactics to ETL/ELT data between the two systems. Lack of consistency: Companies may often find it difficult to keep their data lake and data warehouse architecture consistent.
